Biostatistics Guide for University Students
As a university student diving into biostatistics, you’re stepping into a world where maths powers medical discoveries. You’ll use statistical measures like the mean and median to uncover patterns in genetics, disease spread, and more. Biostatistics not only helps in analysing complex data, but also in designing clinical trials and making evidence-based decisions. Understanding descriptive and inferential statistics, along with regression analysis, becomes your toolbox for advancing health sciences. Mastering statistical software is key for your research journey. As you explore further, you’ll find more about how these techniques can define your future in science and healthcare. Keep going to uncover how.
In short,
- Understand basic statistical measures like mean, median, and standard deviation for data analysis.
- Learn the principles of inferential statistics for hypothesis testing and model building.
- Master statistical software and tools for analysing biological data effectively.
- Grasp the importance of designing experiments with statistical principles to ensure reliable results.
- Explore advanced techniques such as regression and survival analysis for in-depth biological research.
Introduction to Biostatistics
You’re about to delve into how biostatistics is fundamental in biological research, highlighting its essential role. Mastering key concepts and terminology will furnish you with the necessary skills to comprehend and utilise statistical methods efficiently. You’ll also understand why data is central to biostatistical analysis, fostering insights and breakthroughs in the field.
Biostatistics is critical for interpreting complex data, enabling researchers to draw meaningful conclusions about biological phenomena. This discipline applies statistical principles to a wide range of topics in biology, including genetics, epidemiology, and ecological forecasting. By applying these methods, scientists can quantify the effects of various factors on biological systems, providing a robust framework for testing hypotheses and making predictions.
Understanding the basics of biostatistics requires familiarity with statistical measures such as mean, median, and mode, as well as concepts like variance, standard deviation, and confidence intervals. These tools help researchers to describe and analyse the variability within their data, assess the reliability of their findings, and make informed decisions about the significance of their results.
Data collection and analysis are at the forefront of biostatistical work. Properly designed experiments and observational studies are vital for acquiring data that accurately reflects the phenomena under investigation. Statistical software plays a crucial role in analysing this data, offering sophisticated techniques for exploring relationships between variables, identifying trends, and testing theories.
The Role of Biostatistics in Biological Research
Biostatistics plays a crucial role in biological research, enabling scientists to analyse complex data and achieve reliable results. Integrating statistical analysis into your research is not just about working with numbers; it involves recognising patterns that could lead to significant progress in biology and healthcare.
This discipline allows for the development of robust research methodologies, thorough assessment of results, and assurance that the findings can genuinely benefit others. With biostatistics, you are equipped to navigate through vast amounts of data, using statistical methods to enhance clarity and precision in your research.
Whether you are investigating human genetics or assessing the efficacy of new medical interventions, biostatistics serves as a vital partner in translating data into actionable insights with a profound impact on people’s lives.
Key Concepts and Terms in Biostatistics
Having understood the importance of biostatistics in biological research, it’s time to explore the foundational concepts and terms that support this field. Biostatistics applies statistical methods to the analysis in health sciences. It plays a crucial role in designing clinical trials, ensuring the outcomes are reliable and valid.
The design of research is essential; it establishes the plan for studies, from formulating hypotheses to collecting data. Statistical tests are then used to interpret these findings, enabling researchers to identify patterns or effects among biological variability.
Moreover, survival analysis is a vital component of biostatistics, focusing on time-to-event data, which is essential in studies related to lifespans or disease progression. A thorough grasp of these concepts allows for a significant contribution to research that aims to improve health outcomes.
The Importance of Data in Biostatistical Analysis
At the core of biostatistical analysis, data is the essential element that guides researchers through complex health science questions. In this field, your task goes beyond mere number crunching; it involves utilising data to reveal truths that could dramatically alter public health outcomes. Each piece of data gathered in your research acts as a cornerstone for statistical modelling, enhancing the robustness of your studies and the reliability of your conclusions. Without data, there’s no analysis, no survival studies, and no insights—merely speculation. Acknowledging the central role of data in biostatistics equips you to make a meaningful contribution to research that can advance health policies, treatment approaches, and, ultimately, improve lives. In the sphere of public health, your analyses have the potential to lead policymakers towards enhanced wellbeing for communities.
In adopting UK English spellings and grammar, one must be mindful of the subtle differences between UK and US English, particularly in terms of spelling and the use of certain terms. For example, ‘utilising’ instead of ‘utilizing’, and ‘modelling’ instead of ‘modeling’, align with UK conventions. This level of precision in language choice not only adheres to regional standards but also reflects the meticulousness required in biostatistical analyses.
When conveying complex concepts to an audience with a good educational background, such as A-Level students in the UK, clarity and precision in language are paramount. The use of medium-length sentences, coupled with varied sentence structures, facilitates the detailed explanation of biostatistical methods and their significance in public health research. This approach ensures the reader is engaged and can follow the logical progression of ideas without being overwhelmed by technical jargon or dense paragraphs.
Furthermore, providing context to why data is indispensable in biostatistics helps the reader understand its value beyond the superficial level. Instead of making broad statements about the importance of data, explaining how data underpins statistical models and contributes to evidence-based decisions in public health policies offers a concrete understanding of its role.
Statistical Methods in Biostatistics
Having established a foundational understanding of biostatistics, the subsequent phase involves mastering key statistical methods to refine your analytical capabilities. Commence with descriptive statistics to concisely summarise your data. Progress to inferential statistics to understand hypothesis testing and model construction. The curriculum concludes with a focus on advanced statistical methods such as regression analysis, providing you with the ability for intricate data interpretation.
Begin your study with descriptive statistics, which give a comprehensive overview of your dataset through measures of central tendency and variability. This step is essential for understanding the general characteristics of your data before drawing any inferences.
Inferential statistics follow, a domain where you apply statistical tests to determine if your observations are likely to extend beyond your sample to a larger population. This section is critical for supporting your hypotheses with statistical evidence.
The instruction culminates with an in-depth examination of regression analysis and other advanced techniques. These methods are crucial for identifying relationships between variables, enabling the prediction of outcomes based on existing data.
Each section builds on the previous one, ensuring a structured approach to mastering biostatistics. This progression from fundamental concepts to complex analyses is designed to equip you with a versatile toolkit for statistical analysis in the biological sciences.
Descriptive Statistics: Understanding Data Summaries
Why should you focus on descriptive statistics within biostatistics?
In the field dedicated to serving others, a robust understanding of statistical data is essential. Descriptive statistics represent the initial step towards comprehending raw data. By summarising and organising this data, a deeper insight into the scientific and mathematical principles underlying it is obtained.
This process facilitates the presentation of results in a manner that is both clear and succinct, making your findings accessible and actionable. Whether one is engaged in public health, clinical trials, or epidemiology, the ability to communicate complex data effectively is crucial.
Mastering descriptive statistics not only involves analysing numbers; it establishes the basis for making well-informed decisions that have a profound impact on the communities you aim to assist.
Inferential Statistics: From Hypothesis Testing to Model Building
Delving into the field of biostatistics introduces you to inferential statistics, a method that extends past simple data description to allow for hypothesis testing and model construction. This branch of biostatistics enables you to predict and infer outcomes from sample data, applying these findings to broader populations. Through the process of hypothesis testing, you validate assumptions or theories within your study, a fundamental aspect of quantitative research.
Meanwhile, constructing models in statistics permits the development of frameworks predicting outcomes and clarifying the relationships between various variables. Both processes are pivotal in enhancing your research methods, improving patient outcomes, and influencing public health policies. Mastery of these statistical techniques allows you to transform data analysis into practical insights, an essential skill for those committed to advancing science for the benefit of others.
In mastering these statistical methods, you develop the ability to transform data analysis into practical insights, which is essential for those committed to advancing science for the benefit of others.
Advanced Statistical Techniques: Regression Analysis and Beyond
Building on the foundation of inferential statistics, this discussion progresses to advanced statistical techniques, starting with regression analysis, a fundamental approach in biostatistics. For university students with a passion for contributing to methodological research, acquiring proficiency in regression analysis and other advanced methods is crucial. These techniques allow for a deeper examination of data, revealing relationships that are crucial in informing more effective healthcare decisions and interventions.
Beyond regression analysis, there are various statistical methods to tackle complex biostatistical challenges. Engaging with these advanced analyses paves the way for impactful research, positioning you to make significant contributions to the field of biostatistics. The journey through these advanced techniques isn’t solely about achieving mastery in analysis; it’s about using data to support and enhance the well-being of individuals.
As you progress, be aware that applying these statistical methods requires a clear understanding of their principles and the contexts in which they are most effective. For example, regression analysis, in its various forms, enables the examination of the relationship between multiple variables. This is particularly useful in studies aiming to determine potential causative factors for specific health outcomes.
In addition to regression analysis, you will come across techniques such as survival analysis, which is crucial in assessing the time until an event of interest occurs, and multivariate analysis, which examines multiple outcomes simultaneously. Each of these methods offers a unique perspective through which to view biostatistical data, providing insights that are crucial for advancing medical research and practice.
Understanding the complexity and usefulness of these advanced statistical techniques is essential for those aspiring to make a mark in the field of biostatistics. Through dedicated study and application of these methods, you will be well-equipped to design and conduct research that contributes to the improvement of healthcare outcomes. This requires not only a solid grasp of statistical theory but also the ability to apply this knowledge effectively in real-world situations.
Biostatistics in Practice
Having mastered the fundamentals, it’s time to apply biostatistics in practical scenarios. This involves the design of biological experiments as well as the analysis and interpretation of data, marking the juncture where theoretical knowledge is applied in practical settings. Additionally, this section will cover the statistical software and tools that are indispensable for biologists, ensuring you’re fully prepared for your scientific endeavours.
Biostatistics plays a pivotal role in the scientific process, from hypothesis formulation to experimental design, data collection, analysis, and interpretation. Accurate use of statistical methods is critical for drawing reliable conclusions from experimental data.
Experiment design is the first step, where biostatistics aids in structuring the study to ensure valid, reliable, and unbiased results. This includes determining the sample size, which is vital for achieving statistically significant results without unnecessarily using resources or subjects.
Data analysis comes next, where statistical software becomes invaluable. Programs such as R, SAS, and SPSS are extensively used for their powerful capabilities in managing large datasets, performing complex analyses, and visualising data trends and patterns.
Interpretation of statistical outputs requires a deep understanding of biostatistical concepts to make sense of the numerical results. This involves assessing the significance of findings, considering the potential for error, and understanding the implications of the study’s results in the broader context of existing scientific knowledge.
To navigate through these stages effectively, a robust grasp of biostatistical principles and the ability to use statistical software are imperative. This ensures not only the integrity of the research process but also the advancement of scientific knowledge through the rigorous analysis of biological data.
Designing Biological Experiments with Statistical Principles
Integrating biostatistics from the outset is fundamental for conducting biological experiments that yield reliable and insightful outcomes. During the design phase, researchers are tasked with devising a methodology that aligns seamlessly with their research aims, meticulously planning to gather the necessary data for effective analysis in response to their scientific inquiries.
This methodical approach not only streamlines the experimental process but also bolsters the credibility of the findings. Employing statistical principles from the early stages guarantees robust analysis and valid interpretations of the data. A meticulously crafted design stands as the cornerstone of impactful research, offering significant benefits to the scientific community and beyond.
Familiarity with UK English spellings and grammar is essential in this context, ensuring the communication of complex ideas is clear and understandable. The instructional tone adopted here aims to guide the reader through the nuances of experimental design with precision, adhering to academic standards suitable for a UK secondary school or A-Level audience.
In structuring this guidance, sentences of varying lengths are utilised to convey ideas effectively, employing commas for clarity and avoiding overused phrases to maintain the reader’s engagement. By providing context and employing thoughtful transitions, the narrative flows naturally, enhancing the reader’s comprehension of the subject matter.
Data Analysis and Interpretation in Biology
Immersing oneself in data analysis and interpretation unveils the core of biostatistics in practice, showcasing insights crucial for advancing biological research. In the field of biology, the task involves deciphering complex, quantitative data sets. This process transcends mere number crunching to understand the narrative behind the data. The work plays a pivotal role, contributing to breakthroughs with the potential to alter lives.
Statistical Method | Application in Biology | Benefit |
---|---|---|
T-tests | Comparing means | Pinpoints significant distinctions |
ANOVA | Analysis of multiple groups | Identifies sources of variance |
Regression | Modelling relationships | Forecasts trends and patterns |
Chi-square tests | Categorical data analysis | Evaluates associations |
Engaging with data in this manner not only requires a keen eye for detail but also a deep understanding of statistical methods and their applications within biology. This approach empowers researchers to extract meaningful conclusions from data, laying the groundwork for scientific advancements. The ability to accurately interpret data stands at the forefront of biological research, underscoring the significance of biostatistics as an indispensable tool in the scientific community.
Common Statistical Software and Tools for Biologists
In the field of biostatistics, it is essential for biologists to become proficient in using different statistical software and tools to effectively analyse and interpret complex data sets. These tools are fundamental in modern biological research, allowing scientists to apply their expertise to innovative projects that advance science and contribute to healthcare and medicine. By familiarising yourself with these resources, you are not just enhancing your technical skills; you are getting ready to make a valuable contribution to the field of science. Whether it’s for analysing genetic sequences, modelling population dynamics, or researching disease spread, these tools facilitate significant breakthroughs. Incorporating them into your biology career will greatly enhance your ability to make a difference in the world.
By mastering these tools, biologists can apply robust statistical methods to their research, ensuring the accuracy and validity of their results. This knowledge is valuable not only in academic research but also in various industries such as pharmaceuticals, environmental conservation, and public health, where making decisions based on data is crucial.
Understanding how to use these statistical tools requires a commitment to lifelong learning and adaptability, given the fast-paced advancements in technology and methodology. For biologists, this involves staying up to date with the latest software upgrades and analytical techniques, participating in workshops and training programmes, and engaging with the scientific community to share knowledge and experiences.
The Future of Biostatistics in Biology
As one investigates the future of biostatistics in biology, one will find impressive trends and innovations that are changing the ways in which data is analysed.
The increase in significant data volumes in biological sciences emphasises its growing importance, offering a broad scope for new discoveries.
For biology graduates, this development signals various career routes and opportunities in biostatistics, suggesting a dynamic and influential future ahead.
Trends and Innovations in Biostatistical Analysis
Emerging trends and innovations in biostatistical analysis are changing the future of biology, providing new insights and refining research methodologies. As you explore this field, you will see how new methodological advances allow us to tackle various and intricate problems more effectively. These developments not only expand our understanding but also give you the tools to make a significant contribution.
Take into account these innovations:
- Machine Learning Techniques: Developing predictive models significantly enhances the diagnosis and treatment outcomes of diseases.
- Genomic Data Analysis: Using vast amounts of genetic information to pinpoint the origins of diseases and create targeted therapies.
- Environmental Biostatistics: Examining the impact of environmental factors on public health, resulting in improved prevention strategies and healthier communities.
These advancements place you on the forefront of biological research, helping to build a healthier world.
The Increasing Significance of Big Data in Biological Sciences
The role of Big Data in the biological sciences is transforming the approach researchers take to understand complex biological systems, providing insights and opportunities previously unattainable. For a university student aiming to contribute to this field, understanding the significance of Big Data is fundamental. It goes beyond managing substantial data volumes; it’s about unlocking possibilities to address critical biological questions. The incorporation of Big Data into the biological sciences is altering research methodologies, facilitating more accurate and predictive models of biological functions. This shift highlights the necessity for developing strong data analysis capabilities. By honing these skills, you not only propel your career forward but also play a part in paving the way for data-driven breakthroughs that could offer innovative solutions to global health and environmental challenges.
In adapting to UK English, it’s important to pay attention to spelling and grammatical nuances that distinguish it from other forms of English. For instance, words like ‘realize’ become ‘realise’, and ‘modeling’ becomes ‘modelling’. Additionally, maintaining an instructional and authoritative tone is key to effectively communicating with an audience at the A-Level or an advanced reader stage. This involves structuring sentences that are clear, concise, and vary in length to keep the reader engaged while ensuring the information is presented in a logically structured manner.
Careers and Opportunities in Biostatistics for Biology Graduates
A variety of rewarding careers in biostatistics are available to graduates with a degree in biology, opening doors to significant contributions in biological research and advancements. With such a qualification, you’re more than a former student; you’re a trailblazer ready to utilise your rigorous academic training to address real-world challenges. Here are ways you can contribute:
- Engage in a PhD programme to spearhead innovative research.
- Utilise your expertise in public health to tackle global health issues.
- Collaborate with academic or governmental research groups to influence policy and foster innovation.
Opting for a school or programme that matches your desire to contribute to the welfare of others will ensure your career in biostatistics isn’t only fulfilling but also plays a crucial role in creating a healthier, better-informed society. Let’s commence this journey together.
To further enhance your studies, check out our Bioinformatics Guide for University Students available on another page. It’s an invaluable resource for university students eager to dive into the intersection of biology and data analysis.
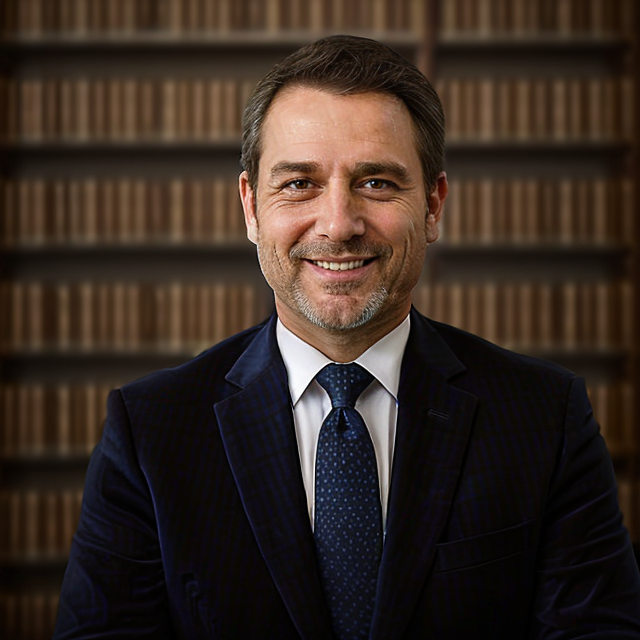
Benjamin Margate is a British biology author, educator and podcaster, leveraging a leveraging a BSc in Biological Sciences from University of Birmingham and over 10 years of expertise creating and curating detailed biology content. His work focuses on providing educational blogs and resources for biology students and facilitating connections with professional tutors. Recognised for his extensive knowledge and commitment to biology education, Benjamin’s contributions have become a cornerstone for learners seeking to enhance their understanding of the subject.